Luc Anselin, a Fellow of the University Consortium for Geographic Information Science, recently remarked that "GIScience [is] morphing into spatial data science” (Anselin 1027).
Is it really?
Depending on the origin stories you choose, both GIScience and Data Science began to take shape in the 1960s and 70s. Stanford professor David Donoho traces the origins of Data Science to the work of the maverick statistician John Tukey, then Donoho’s undergraduate thesis adviser at Princeton (and one of my own scholar-heroes; hence my choice of stories).
Donoho’s definition of data science as “a superset of the fields of statistics and machine learning which adds some technology for ‘scaling up’ to ‘big data’” belies his skepticism about the hype that surrounds the “contemplated field.” Indeed, Gartner reports that data science and machine learning began reaching the peak of their “hype cycle” in the past year.
Concerns about predicted shortfalls of qualified practitioners have led organizations like the
National Academies of Science, the
National Science Foundation, and the
National Institutes of Health to charge distinguished panels to develop strategic plans for Data Science, including data science education. These reports barely mention geospatial data and methods, and certainly don’t recognize GIScience as an integral part of Data Science.
Anselin (2017) might attribute this to “space skepticism,” the tendency of mainstream scientists not to consider spatial thinking “fundamental to the scientific process itself.” In our own higher education outreach, my colleagues in Esri’s Education team and I have noted a widespread belief among scientists beyond GIScience that spatial data are “just another data type."
Beyond the academy, there is evidence of convergence in the occupations as well. A search on “data scientist” at O*NET Online – the U.S. Department of Labor’s database of occupations – produces "Geospatial Information Scientists and Technologists" and "Remote Sensing Scientists and Technologists" among its top ten search results.
First 20 occupations associated with the search term "data scientist" at the U.S. Department of Labor's O*Net OnLine web site.(One might wonder, why does the Department of Labor database not include an occupation called "data scientist"? One explanation is that GIScience's hype cycle peaked much earlier – arguably in 2003, when the U.S. Department of Labor highlighted “Geospatial Technology” as a high-growth technology industry. Advocacy for formal occupations crescendoed soon thereafter.)
The recent events hosted by Harvard's Center for Geographic Analysis, and soon by CaGIS and UCGIS, may reflect a widening interest in the intersection of GIScience and Data Science - among GIScientists, at least. Harvard's event attracted 26 presenters and panelists representing academic institutions, government agencies, and industry, and a record-high registration of over 250 participants in total. Organizers Matt Wilson (Professor Geography, University of Kentucky, and Visiting Scholar, Harvard), Wendy Guan (Executive Director, Harvard CGA), and I aimed to bring together mainstream data scientists and GIScientists, to review the status of both fields, and to explore commonalities.
Keynoter
Francesca Dominici (Professor Biostatistics, co-chair Harvard’s Data Science Initiative) described a research study that applied a neural network to predict a continuous, 1 km grid of daily air pollution levels across the continental U.S.. Fused with claims records for over 67 million Medicare patients, the
research suggests that there is no “safe” level of fine particulate matter pollution (produced primarily by fossil-fueled power plants) for senior citizens.
In a panel themed “Sensors, Smart Objects and Infrastructure for Data Science,"
Carlo Ratti (Director, SENSEable Cities Laboratory, MIT) focused his short presentation on a project called
TrashTrack, which addresses the research question, “why do we know so much about the supply chain and so little about the ‘removal chain'?” The project mobilized volunteers in Seattle who attached small, cheap, location-aware sensors (designed in Ratti's Lab) to 3,000 trash objects. The visualized trajectories of tracked trash revealed far-flung, nationwide removal chains, and raised new questions about environmental justice.
In the same session,
Brendan Meade (Professor Earth & Planetary Sciences and Affiliate in Computer Science, Harvard), discussed how machine learning is changing the condition of possibility of earthquake prediction, and reported progress in using neural networks to predicting where aftershocks will occur.
A second panel titled "Crowdsourcing, Geocomputation, and Spatiotemporal Analysis" included
Amen Mashariki (Urban Analytics program lead, Esri). Amen reflected on his former role as chief data scientist for the City of New York, and pointed out the prevalence of predictive policing in U.S. cities. Emphasizing the need for transparency in prediction algorithms, he described an
outreach strategy to promote public understanding of algorithms in his new home, the City of Baltimore.
Alex Singleton (Professor of Geographic Information Science and Director of the University of Liverpool’s Geographic Data Science Lab) explained why traditional sources of social science data are under threat, including national censuses and large-scale social surveys. Emergent new data sources are challenging traditional modes of inquiry.
In a third panel on "Data Science for Cities, Health and Environment,"
Björn Menze (Professor Computer Science, TU München) presented work on algorithm design for medical image processing, including CT Scans. Noting that hundreds of thousands of such images are available for analysis at national health information repositories, he demonstrated how machine learning enables new mappings of disease patterns.
(Menze’s work came up earlier in the day, in a different context. Our host for the event,
Jason Ur, (Professor Archeology and CGA Faculty Director) mentioned in his introductory remarks that Björn used similar algorithms to detect thousands of archaeological sites in remotely-sensing imagery
– discoveries that would have taken Jason years to uncover through traditional field methods.)
Michael Goodchild (Professor Emeritus Geography, UC Santa Barbara) offered a second keynote address entitled "The Landscape of GIScience." Goodchild, who coined the term “Geographic Information Science” in 1992, wondered if the name "Data Science" isn't "retrograde," given that "information is data fit for purpose." Still, he agreed that rise of data science does provide opportunities for GIScience. "Carpe diem," Mike concluded.
Should GIScience converge with Data Science?
Allowing that some evidence supports Anselin's claim that GIScience is morphing into spatial data science, a second question remains: should it? Answers will vary depending on one's viewpoint and values. I'm an educator first and foremost, and my primary sense of duty is for my students' success – before and after they graduate. From that perspective I think about spatial data science in context of the evolution of work in an age of automation.
I hear a growing chorus of economists, tech leaders, and forward-looking historians anticipate fundamental disruption of traditional employment by increasingly capable machines. Management consultants Richard and Daniel Susskind, authors of The Future of the Professions (2016, p), foresee that “in the long run, increasingly capable machines will transform the work of professionals … leaving most … to be replaced by less expert people and high-performing systems.” Kelleher and Tierney (2018, 67), for example, suggest that "data science is best understood as a partnership between a data scientist and a computer."
Recognizing that the outsourcing of work to machines is nothing new, and that observers are notoriously bad at anticipating the new jobs that disruptive technologies eventually create, the Susskinds don’t predict future occupations that may replace the traditional professions. Instead, they suggest twelve future roles for which education should help people prepare. Those roles are:
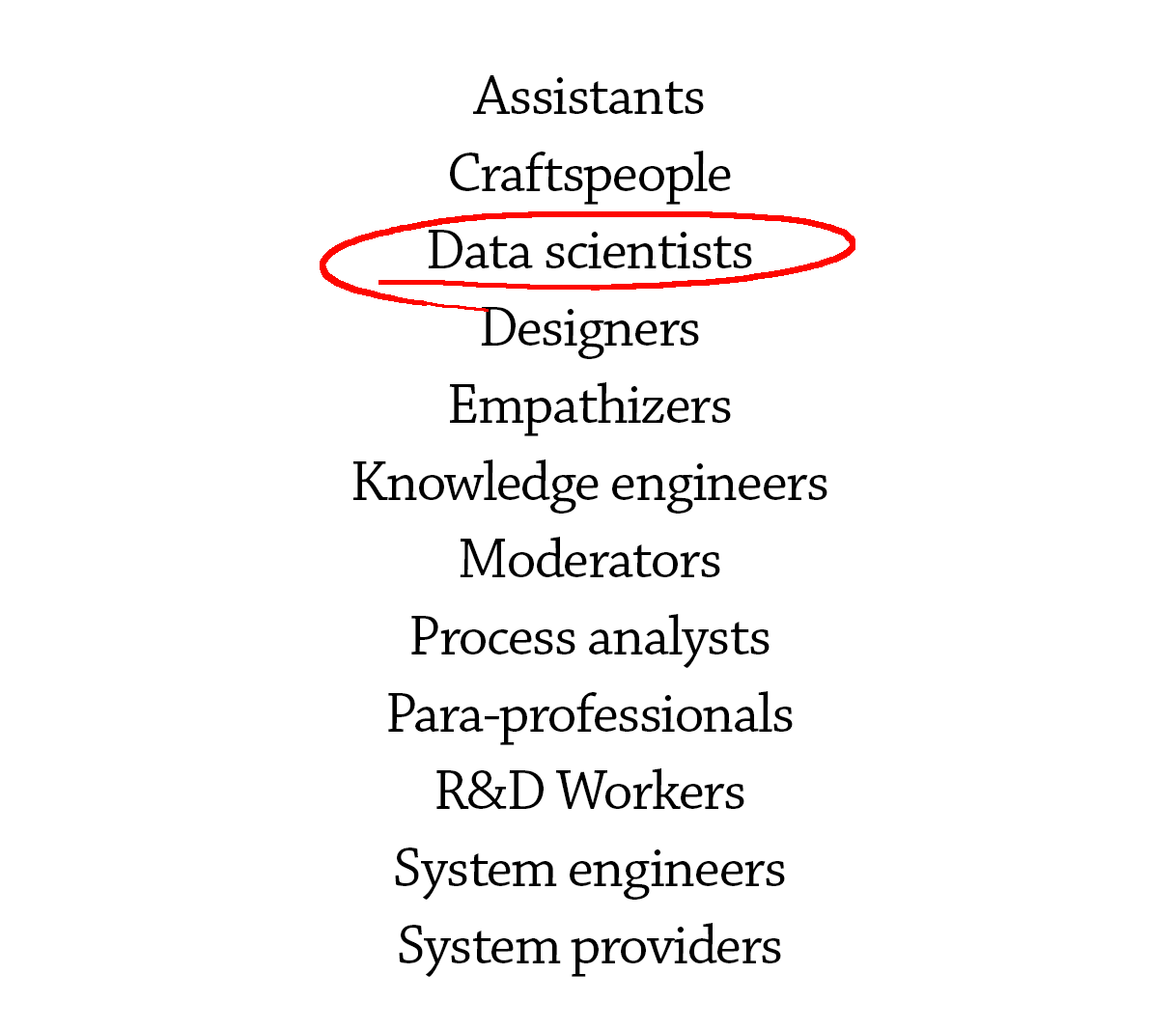
Future roles in a post-professional economy (Susskind and Susskind 2015)
As the search results of the Department of Labor's O*Net database (above) suggest, "data scientist" is a role that workers in many occupations will be expected to play. I, for one, am becoming convinced that graduates of GIS-related degree and certificate programs should be prepared to play that role, to a greater extent than their predecessors already do.
One implication is that tomorrow’s spatial data scientists – professionals with specialized competence with georeferenced data “wrangling,” analysis, visualization, and story telling – will need skills and abilities that span all three industry sectors of the Department of Labor's
Geospatial Technology Competency Model: positioning and data acquisition, analysis and modeling, and software and app development. A corollary to that point is the need for future revisions of the GTCM to incorporate data science skills and technologies, including machine learning techniques and greater emphases on statistics and programming.
While GIScience may be "morphing into spatial data science," the fact remains that few data scientists recognize that spatial data are special, as Goodchild first argued in 1992. However, that "space skepticism" may yet be overcome by "successful use cases ... demonstrating indisputable business advantages" and "unequivocal evidence that the incorporation of an explicit spatial perspective leads to better solutions..." (Anselin 2017).
Carpe diem indeed!
Berman, Francine, Rob Rutenbar, Brent Hailpern, Henrik Christensen, Susan Davidson, Deborah Estrin, Michael Franklin, Margaret Martonosi, Padma Raghavan, Victoria Stodden, and Alexander S. Szalay (2018) Realizing the Potential of Data Science. Communications of the ACM, 61:4, 67-72. Donoho, David (2017). 50 Years of Data Science. Journal of Computational and Graphical Statistics, 26(4), 745-766.
Kelleher, John D., and Brendan Tierney (2018). Data Science. MIT Press Essential Knowledge series.
Susskind, Richard E., and Daniel Susskind (2015). The Future of the Professions: How Technology will Transform the Work of Human Experts. Oxford University Press.